Thanks to the continued development of richer and ever more sophisticated sets of encoding tools, it has been possible to significantly increase the efficiency of each new generation of video codecs. For content creators, video encoding can be the most critical part of a workflow. In essence, the video encoder has one mission; to optimize the video quality and bitrate. Good broadcast video encoders need to make good decisions in real-time.
To date, constant hardware developments have partially fulfilled the ever-increasing requirements in processing power. However, simultaneous increases in resolution and frame rates mean reliance upon hardware improvements is just not enough for certain applications. It means encoder developers often need to compromise on the encoding tools adopted to meet the computational resources budget for a given application. As a result, some of the encoder designs that have been developed have delivered a compression efficiency that falls short of the codec’s overall potential.
The optimization of AI and Machine Learning
Over the past few years, our video research experts have invested large amounts into maximizing opportunities from recent developments in Artificial Intelligence (AI) and Machine Learning (ML), as part of our continued commitment to push the boundaries and deliver industry-leading video quality and hardware efficiency. Optimized ML and AI can be used to help guide encoding tool selection for all use cases while guaranteeing the most efficient use of processing power.
We believe the use of ML and AI form the key to reducing infrastructure costs and improving the overall viewing experience. In recent months, I’ve described the benefits of MediaKind’s groundbreaking AI-based Compression Technology (ACT). But we have also driven innovations in other areas of compression delivery, including ML-guided CTU splitting, Constant Video Quality (CVQ) Adaptive Bitrate Streaming (ABR), and ML-Based video up-conversion.
In video encoding, AI can be used to understand the characteristics of video content and then map how the encoders use their processing options to achieve the best results. AI helps analyze decisions made by encoders using different toolsets. This makes it possible to identify complex data patterns that predict the optimal tools or encoding options to use in any given scenario. It means we can better understand the dependency between those tools and the content.
Through this analysis, we can generate AI-driven decisions within the encoder. We can then use AI-driven compression to adaptively apply all available compute resource using the most beneficial balance between the possible encoding features. Doing so helps ensure the processing power is spent where it is most effective for that type of content. It proves far more accurate than any human-defined heuristic or algorithm could.
Using Machine Learning to infer Coding Unit splitting for HEVC
MediaKind has launched a new application paper titled ‘Improving Video Compression with AI: Using Machine Learning to infer Coding Unit splitting for HEVC.’ This paper aims to demonstrate how it’s now possible to extend the use of ML techniques beyond previous methods, such as adjusting traditional encoder parameters (such as the search range for motion estimation) or guiding the choice of encoding modes, predictions, and in particular, block splitting.
By block splitting, we refer to how the input pictures in a frame are split into fixed-sized blocks. Each block is spatially and/or temporally predicted based on its causal neighbors. The resulting residue is then encoded using a transform, quantization, and entropy encoding approach.
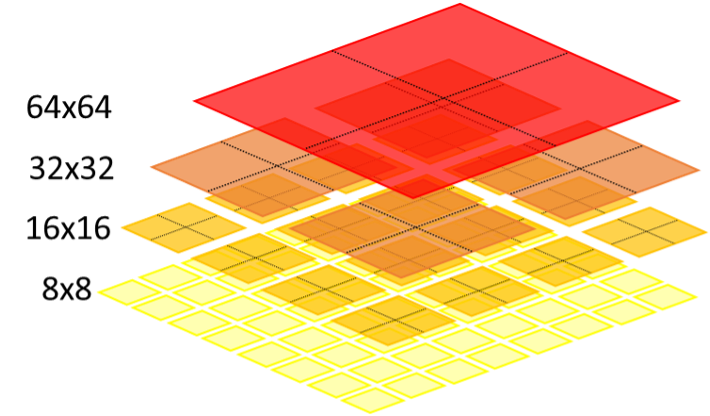
Just like its predecessors MPEG-2 and MPEG-4, HEVC is also block-based hybrid video coded. But unlike its predecessors, HEVC can be delivered in a wider range of block units than the traditional 16×16. Delivered through Coding Tree Unit (CTU), the blocks sizes include 16×16 to 32×32 and 64×64. It increases coding efficiency by adjusting the prediction and transforming sizes to the local nature of the content.
However, it comes at the cost of a significant increase in computation, as more options are required to evaluate the order to determine the optimal choice. For accurate prediction, the ability to determine block sizes likely to be used before encoding would mean only a subset of all possible options would need to be evaluated by the main encoder, with a negligible effect in compression efficiency. Since the optimization loop contributes to most codec complexity, this approach can potentiate huge computational savings.
Download MediaKind’s new Innovation Paper!
So, what does that mean for the future? In our latest research, we address the economic advantages of using ML to help guide CTU splitting. The reasons are numerous, ranging from enabling operators to run larger numbers of channels in the same computational resources, or the ability to use a lower performance, less expensive hardware option to achieve an equivalent compression efficiency. ML-guided CTU splitting enables AI to refine individual areas of the codec for each specific application. The bottom line? This approach aligns with our overall strategy to deliver the most efficient video coding solutions while minimizing energy costs.
To learn more about our research and why MediaKind believes a new generation of AI-powered encoders are helping to raise the bar on codec efficiency for the entire encoder industry, download our innovation paper today.